ARTIFICIAL INTELLIGENCE (AI) - MACHINE LEARNING (ML) - DEEP LEARNING (DL)
ARTIFICIAL INTELLIGENCE (AI) - MACHINE LEARNING (ML) - DEEP LEARNING (DL)
This text discusses the topics of Artificial Intelligence (AI), Machine Learning (ML), and Deep Learning (DL).
AI, ML, and DL are interconnected technologies for creating intelligent systems that learn from data to make decisions. AI encompasses a wide scope of human-like tasks, while ML focuses on data-driven learning. DL, a subset of ML, utilizes deep neural networks for complex pattern recognition. These technologies collectively enhance problem-solving across diverse domains. Artificial intelligence (AI), machine learning (ML), and deep learning (DL) are all related technologies that can be used to create intelligent systems. These systems can learn from data and make decisions based on that learning, without being explicitly programmed. AI, ML, and DL are all powerful tools that can be used to solve complex problems and improve many different aspects of our lives.
In today’s ever-evolving world, new technologies designed to make our lives easier are constantly being introduced. One of these critical pieces of technology is AI or artificial intelligence. You may be familiar with AI’s most common form, digital assistants, which you can find on your phone (ex., Siri) and in your home (ex., Amazon Alexa).
But what is artificial intelligence? How does artificial intelligence relate to machine learning and deep learning? Are they the same? Are the terms used interchangeably or unrelated?
Artificial Intelligence is often used as a catch-all term for machine learning and deep learning. However, there are many differences between these types of AI, so it’s essential to learn what each term represents and the differences/relationships they share.
Let’s start by looking at some basic definitions of these terms:
Artificial Intelligence (AI): Developing machines to mimic human intelligence and behaviour.
Machine Learning (ML): Algorithms that learn from structured data to predict outputs and discover patterns in that data.
Deep Learning (DL): Algorithms based on highly complex neural networks that mimic the way a human brain works to detect patterns in large unstructured data sets.
Artificial Intelligence:
Artificial Intelligence is basically the mechanism to incorporate human intelligence into machines through a set of rules(algorithm). AI is a combination of two words: “Artificial” meaning something made by humans or non-natural things and “Intelligence” meaning the ability to understand or think accordingly. Another definition could be that “AI is basically the study of training your machine(computers) to mimic a human brain and its thinking capabilities”.
AI focuses on 3 major aspects(skills): learning, reasoning, and self-correction to obtain the maximum efficiency possible.
Machine Learning:
Machine Learning is basically the study/process which provides the system(computer) to learn automatically on its own through experiences it had and improve accordingly without being explicitly programmed. ML is an application or subset of AI. ML focuses on the development of programs so that it can access data to use it for itself. The entire process makes observations on data to identify the possible patterns being formed and make better future decisions as per the examples provided to them. The major aim of ML is to allow the systems to learn by themselves through experience without any kind of human intervention or assistance.
Deep Learning:
Deep Learning is basically a sub-part of the broader family of Machine Learning which makes use of Neural Networks(similar to the neurons working in our brain) to mimic human brain-like behavior. DL algorithms focus on information processing patterns mechanism to possibly identify the patterns just like our human brain does and classifies the information accordingly. DL works on larger sets of data when compared to ML and the prediction mechanism is self-administered by machines.
Below is a table of differences between Artificial Intelligence, Machine Learning and Deep Learning
Difference Between AI vs ML vs DL
Artificial Intelligence | Machine Learning | Deep Learning |
---|---|---|
AI stands for Artificial Intelligence, and is basically the study/process which enables machines to mimic human behaviour through particular algorithm. | ML stands for Machine Learning, and is the study that uses statistical methods enabling machines to improve with experience. | DL stands for Deep Learning, and is the study that makes use of Neural Networks(similar to neurons present in human brain) to imitate functionality just like a human brain. |
AI is the broader family consisting of ML and DL as it’s components. | ML is the subset of AI. | DL is the subset of ML. |
AI is a computer algorithm which exhibits intelligence through decision making. | ML is an AI algorithm which allows system to learn from data. | DL is a ML algorithm that uses deep(more than one layer) neural networks to analyze data and provide output accordingly. |
Search Trees and much complex math is involved in AI. | If you have a clear idea about the logic(math) involved in behind and you can visualize the complex functionalities like K-Mean, Support Vector Machines, etc., then it defines the ML aspect. | If you are clear about the math involved in it but don’t have idea about the features, so you break the complex functionalities into linear/lower dimension features by adding more layers, then it defines the DL aspect. |
The aim is to basically increase chances of success and not accuracy. | The aim is to increase accuracy not caring much about the success ratio. | It attains the highest rank in terms of accuracy when it is trained with large amount of data. |
Three broad categories/types Of AI are: Artificial Narrow Intelligence (ANI), Artificial General Intelligence (AGI) and Artificial Super Intelligence (ASI) | Three broad categories/types Of ML are: Supervised Learning, Unsupervised Learning and Reinforcement Learning | DL can be considered as neural networks with a large number of parameters layers lying in one of the four fundamental network architectures: Unsupervised Pre-trained Networks, Convolutional Neural Networks, Recurrent Neural Networks and Recursive Neural Networks |
The efficiency Of AI is basically the efficiency provided by ML and DL respectively. | Less efficient than DL as it can’t work for longer dimensions or higher amount of data. | More powerful than ML as it can easily work for larger sets of data. |
Examples of AI applications include: Google’s AI-Powered Predictions, Ridesharing Apps Like Uber and Lyft, Commercial Flights Use an AI Autopilot, etc. | Examples of ML applications include: Virtual Personal Assistants: Siri, Alexa, Google, etc., Email Spam and Malware Filtering. | Examples of DL applications include: Sentiment based news aggregation, Image analysis and caption generation, etc. |
AI refers to the broad field of computer science that focuses on creating intelligent machines that can perform tasks that would normally require human intelligence, such as reasoning, perception, and decision-making. | ML is a subset of AI that focuses on developing algorithms that can learn from data and improve their performance over time without being explicitly programmed. | DL is a subset of ML that focuses on developing deep neural networks that can automatically learn and extract features from data. |
AI can be further broken down into various subfields such as robotics, natural language processing, computer vision, expert systems, and more. | ML algorithms can be categorized as supervised, unsupervised, or reinforcement learning. In supervised learning, the algorithm is trained on labeled data, where the desired output is known. In unsupervised learning, the algorithm is trained on unlabeled data, where the desired output is unknown. | DL algorithms are inspired by the structure and function of the human brain, and they are particularly well-suited to tasks such as image and speech recognition. |
AI systems can be rule-based, knowledge-based, or data-driven. | In reinforcement learning, the algorithm learns by trial and error, receiving feedback in the form of rewards or punishments. | DL networks consist of multiple layers of interconnected neurons that process data in a hierarchical manner, allowing them to learn increasingly complex representations of the data. |
The Relationship Between AI, ML and DL
To begin, we’re going to start with the relationship between Artificial Intelligence (AI), Machine Learning (ML) and Deep Learning (DL). Since these three elements are interconnected (meaning you can’t have one without the other), exploring their relationship is vital before we break down each factor individually.
Machine Learning is a sub-category of AI, and Deep Learning is a sub-category of ML, meaning they are both forms of AI.
Artificial intelligence is the broad idea that machines can intelligently execute tasks by mimicking human behaviours and thought processes.
Machine learning, a subset of AI, revolves around the idea that machines can learn and adapt through experiences and data to complete specific tasks. An example would be predicting the weather forecast for the next seven days based on data from the previous year and the previous week. Every day, the data from the previous year/week changes, so the ML model must adapt to the new data.
Deep learning is a subset of ML. DL models are based on highly complex neural networks that mimic how the brain works. With many layers of processing units, deep learning takes it a step further to learn complex patterns in large amounts of data. For example, deep learning (combined with computer vision) in a driverless car can identify a person crossing the road.
Artificial Intelligence:
Reactive Machines AI: These are AI systems that operate based on pre-programmed rules and respond to specific inputs. They don't have the ability to learn or adapt from experience. Chess-playing computers in the early days of AI are examples of reactive machines.
Limited Memory AI: These systems can learn from historical data to make informed decisions and improve their performance over time. They have a limited ability to adapt to new situations but still lack full learning capabilities. Self-driving cars often incorporate limited memory AI.
Theory of Mind AI: This is a hypothetical form of AI that can understand human emotions, intentions, beliefs, and consciousness. It can attribute mental states to itself and others, enabling it to interact with humans in a more socially aware manner.
Self-aware AI: This is an advanced form of AI that possesses consciousness and self-awareness, understanding its own existence and potentially having subjective experiences. This level of AI remains largely in the realm of science fiction and philosophical discussions.
Machine Learning:
Supervised Learning: In this type of learning, the model is trained on a labeled dataset, where each input is associated with the correct output. The goal is for the model to learn the mapping between inputs and outputs so that it can make accurate predictions on new, unseen data. Examples include classification and regression tasks.
Unsupervised Learning: Here, the model is given a dataset without labeled outputs. The goal is to find patterns, structures, or relationships within the data. Common tasks include clustering (grouping similar data points) and dimensionality reduction (reducing the number of features while preserving important information)
Reinforcement Learning: This type of learning is inspired by behavioral psychology. An agent learns to interact with an environment to achieve a goal by receiving feedback in the form of rewards or penalties. The agent learns optimal actions through trial and error, aiming to maximize cumulative rewards over time.
Deep Learning:
Convolutional Neural Networks (CNN): Designed for image and video analysis, CNNs are specialized in processing grid-like data such as pixels. They use convolutional layers to automatically learn hierarchical features from images, making them highly effective for tasks like object detection and image recognition.
Recurrent Neural Networks (RNN): RNNs are designed for sequences of data, such as time series or sequences of text. They have loops that allow information to be passed from one step of the network to the next, allowing them to maintain context and handle sequential dependencies. Long Short-Term Memory (LSTM) and Gated Recurrent Unit (GRU) are popular variants of RNNs.
Generative Adversarial Networks (GAN): GANs consist of a generator network and a discriminator network. The generator creates new data instances that aim to be indistinguishable from real data, while the discriminator tries to distinguish between real and generated data. GANs are used for tasks like image and text generation.
Deep Belief Networks (DBN): A Deep Belief Network (DBN) is a specific type of deep learning architecture that consists of multiple layers of latent variables (hidden units) and is primarily used for unsupervised learning tasks, such as generative modeling and feature learning. DBNs were introduced by Geoffrey Hinton and his colleagues in the mid-2000s and have contributed to the advancement of deep learning techniques.
ARTIFICIAL INTELLIGENCE
What is Artificial Intelligence?
Artificial intelligence or AI recreates human intelligence and behaviour using algorithms, data, and models. AI predicts, automates, and completes tasks typically done by humans with greater accuracy and precision, reduced bias, cost, and timesaving.
When is Artificial Intelligence Used?
Artificial intelligence is used when a machine completes a task using human intellect and behaviours. For example, Roomba, the smart robotic vacuum, uses AI to analyze the size of the room, obstacles, and pathways. Just like a human being taking this information into account, Roomba then retains this information and creates the most efficient route for vacuuming.
Application of Artificial Intelligence:-
Artificial Intelligence has various applications in today's society. It is becoming essential for today's time because it can solve complex problems with an efficient way in multiple industries, such as Healthcare, entertainment, finance, education, etc. AI is making our daily life more comfortable and fast.
Following are some sectors which have the application of Artificial Intelligence:
1. AI in Astronomy:
- Artificial Intelligence can be very useful to solve complex universe problems. AI technology can be helpful for understanding the universe such as how it works, origin, etc.
2. AI in Healthcare:
- In the last, five to ten years, AI becoming more advantageous for the healthcare industry and going to have a significant impact on this industry.
- Healthcare Industries are applying AI to make a better and faster diagnosis than humans. AI can help doctors with diagnoses and can inform when patients are worsening so that medical help can reach to the patient before hospitalization.
3. AI in Gaming:
- AI can be used for gaming purpose. The AI machines can play strategic games like chess, where the machine needs to think of a large number of possible places.
4. AI in Finance:
- AI and finance industries are the best matches for each other. The finance industry is implementing automation, chatbot, adaptive intelligence, algorithm trading, and machine learning into financial processes.
5. AI in Data Security:
- The security of data is crucial for every company and cyber-attacks are growing very rapidly in the digital world. AI can be used to make your data more safe and secure. Some examples such as AEG bot, AI2 Platform,are used to determine software bug and cyber-attacks in a better way.
6. AI in Social Media:
- Social Media sites such as Facebook, Twitter, and Snapchat contain billions of user profiles, which need to be stored and managed in a very efficient way. AI can organize and manage massive amounts of data. AI can analyze lots of data to identify the latest trends, hashtag, and requirement of different users.
7. AI in Travel & Transport:
- AI is becoming highly demanding for travel industries. AI is capable of doing various travel related works such as from making travel arrangement to suggesting the hotels, flights, and best routes to the customers. Travel industries are using AI-powered chatbots which can make human-like interaction with customers for better and fast response.
8. AI in Automotive Industry:
- Some Automotive industries are using AI to provide virtual assistant to their user for better performance. Such as Tesla has introduced TeslaBot, an intelligent virtual assistant.
- Various Industries are currently working for developing self-driven cars which can make your journey more safe and secure.
9. AI in Robotics:
- Artificial Intelligence has a remarkable role in Robotics. Usually, general robots are programmed such that they can perform some repetitive task, but with the help of AI, we can create intelligent robots which can perform tasks with their own experiences without pre-programmed.
- Humanoid Robots are best examples for AI in robotics, recently the intelligent Humanoid robot named as Erica and Sophia has been developed which can talk and behave like humans.
10. AI in Entertainment:
- We are currently using some AI based applications in our daily life with some entertainment services such as Netflix or Amazon. With the help of ML/AI algorithms, these services show the recommendations for programs or shows.
11. AI in Agriculture:
- Agriculture is an area which requires various resources, labor, money, and time for best result. Now a day's agriculture is becoming digital, and AI is emerging in this field. Agriculture is applying AI as agriculture robotics, solid and crop monitoring, predictive analysis. AI in agriculture can be very helpful for farmers.
12. AI in E-commerce:
- AI is providing a competitive edge to the e-commerce industry, and it is becoming more demanding in the e-commerce business. AI is helping shoppers to discover associated products with recommended size, color, or even brand.
13. AI in education:
- AI can automate grading so that the tutor can have more time to teach. AI chatbot can communicate with students as a teaching assistant.
- AI in the future can be work as a personal virtual tutor for students, which will be accessible easily at any time and any place.
MACHINE LEARNING
What is Machine Learning?
Machine learning, or ML, is a type of AI that uses algorithms to learn from data to make sense of it or predict a pattern. Machine learning uses methods from neural networks, statistics, operations research, and physics to find hidden insights within data without being programmed where to look or what to conclude. For example, machine learning is used to develop self-learning processing where software is given instructions on accomplishing a specific task. The machine is then trained and learns how to perform the job by analyzing relevant data and algorithms, allowing it to understand how to accomplish the task and then evolve its performance.
When is Machine Learning Used?
Use machine learning when you’re looking to teach a model how to perform a task, such as predicting an output or discovering a pattern using structured data. For example, Spotify builds you a customized playlist based on your favourite songs and the data from other users who share your likes and dislikes.
Applications of Machine Learning:-
Machine learning is a buzzword for today's technology, and it is growing very rapidly day by day. We are using machine learning in our daily life even without knowing it such as Google Maps, Google assistant, Alexa, etc. Below are some most trending real-world applications of Machine Learning:
1. Image Recognition:
Image recognition is one of the most common applications of machine learning. It is used to identify objects, persons, places, digital images, etc. The popular use case of image recognition and face detection is, Automatic friend tagging suggestion:
Facebook provides us a feature of auto friend tagging suggestion. Whenever we upload a photo with our Facebook friends, then we automatically get a tagging suggestion with name, and the technology behind this is machine learning's face detection and recognition algorithm.
It is based on the Facebook project named "Deep Face," which is responsible for face recognition and person identification in the picture.
2. Speech Recognition:
While using Google, we get an option of "Search by voice," it comes under speech recognition, and it's a popular application of machine learning.
Speech recognition is a process of converting voice instructions into text, and it is also known as "Speech to text", or "Computer speech recognition." At present, machine learning algorithms are widely used by various applications of speech recognition. Google assistant, Siri, Cortana, and Alexa are using speech recognition technology to follow the voice instructions.
3. Traffic Prediction:
If we want to visit a new place, we take help of Google Maps, which shows us the correct path with the shortest route and predicts the traffic conditions.
It predicts the traffic conditions such as whether traffic is cleared, slow-moving, or heavily congested with the help of two ways:
- Real Time location of the vehicle form Google Map app and sensors
- Average time has taken on past days at the same time.
Everyone who is using Google Map is helping this app to make it better. It takes information from the user and sends back to its database to improve the performance.
4. Product Recommendations:
Machine learning is widely used by various e-commerce and entertainment companies such as Amazon, Netflix, etc., for product recommendation to the user. Whenever we search for some product on Amazon, then we started getting an advertisement for the same product while internet surfing on the same browser and this is because of machine learning.
Google understands the user interest using various machine learning algorithms and suggests the product as per customer interest.
As similar, when we use Netflix, we find some recommendations for entertainment series, movies, etc., and this is also done with the help of machine learning.
5. Self-Driving cars:
One of the most exciting applications of machine learning is self-driving cars. Machine learning plays a significant role in self-driving cars. Tesla, the most popular car manufacturing company is working on self-driving car. It is using unsupervised learning method to train the car models to detect people and objects while driving.
6. Email Spam and Malware Filtering:
Whenever we receive a new email, it is filtered automatically as important, normal, and spam. We always receive an important mail in our inbox with the important symbol and spam emails in our spam box, and the technology behind this is Machine learning. Below are some spam filters used by Gmail:
- Content Filter
- Header filter
- General blacklists filter
- Rules-based filters
- Permission filters
Some machine learning algorithms such as Multi-Layer Perceptron, Decision tree, and Naïve Bayes classifier are used for email spam filtering and malware detection.
7. Virtual Personal Assistant:
We have various virtual personal assistants such as Google assistant, Alexa, Cortana, Siri. As the name suggests, they help us in finding the information using our voice instruction. These assistants can help us in various ways just by our voice instructions such as Play music, call someone, Open an email, Scheduling an appointment, etc.
These virtual assistants use machine learning algorithms as an important part.
These assistant record our voice instructions, send it over the server on a cloud, and decode it using ML algorithms and act accordingly.
8. Online Fraud Detection:
Machine learning is making our online transaction safe and secure by detecting fraud transaction. Whenever we perform some online transaction, there may be various ways that a fraudulent transaction can take place such as fake accounts, fake ids, and steal money in the middle of a transaction. So to detect this, Feed Forward Neural network helps us by checking whether it is a genuine transaction or a fraud transaction.
For each genuine transaction, the output is converted into some hash values, and these values become the input for the next round. For each genuine transaction, there is a specific pattern which gets change for the fraud transaction hence, it detects it and makes our online transactions more secure.
9. Stock Market Trading:
Machine learning is widely used in stock market trading. In the stock market, there is always a risk of up and downs in shares, so for this machine learning's long short term memory neural network is used for the prediction of stock market trends.
10. Medical Diagnosis:
In medical science, machine learning is used for diseases diagnoses. With this, medical technology is growing very fast and able to build 3D models that can predict the exact position of lesions in the brain.
It helps in finding brain tumors and other brain-related diseases easily.
11. Automatic Language Translation:
Nowadays, if we visit a new place and we are not aware of the language then it is not a problem at all, as for this also machine learning helps us by converting the text into our known languages. Google's GNMT (Google Neural Machine Translation) provide this feature, which is a Neural Machine Learning that translates the text into our familiar language, and it called as automatic translation.
The technology behind the automatic translation is a sequence to sequence learning algorithm, which is used with image recognition and translates the text from one language to another language.
DEEP LEARNING
What is Deep Learning?
Deep learning is the evolution of machine learning and neural networks, which uses advanced computer programming and training to understand complex patterns hidden in large data sets. DL is about understanding how the human brain works in different situations and then trying to recreate its behaviour. Deep learning is used for complicated problems such as facial recognition, defect detection and image processing.
When to Use Deep Learning?
Deep learning is used to complete complex tasks and train models using unstructured data. For example, deep learning is commonly used in image classification tasks like facial recognition. Although machine learning models can also identify faces, deep learning models are more accurate. In this case, it takes the unstructured data (images of faces) and extracts factors such as the various facial features. The extracted features are then matched to those stored in a database.
Applications of Deep Learning:-
Deep learning has found applications across a wide range of fields due to its ability to automatically learn patterns and features from large datasets. Some notable applications of deep learning include
AI vs. Machine Learning vs. Deep Learning Examples:
Examples of Artificial Intelligence:
Artificial Intelligence (AI) refers to the development of computer systems that can perform tasks that would normally require human intelligence.
Here are some examples of Artificial Intelligence:
There are numerous examples of AI applications across various industries. Here are some common examples:
- Speech recognition: speech recognition systems use deep learning algorithms to recognize and classify images and speech. These systems are used in a variety of applications, such as self-driving cars, security systems, and medical imaging.
- Personalized recommendations: E-commerce sites and streaming services like Amazon and Netflix use AI algorithms to analyze users’ browsing and viewing history to recommend products and content that they are likely to be interested in.
- Predictive maintenance: AI-powered predictive maintenance systems analyze data from sensors and other sources to predict when equipment is likely to fail, helping to reduce downtime and maintenance costs.
- Medical diagnosis: AI-powered medical diagnosis systems analyze medical images and other patient data to help doctors make more accurate diagnoses and treatment plans.
- Autonomous vehicles: Self-driving cars and other autonomous vehicles use AI algorithms and sensors to analyze their environment and make decisions about speed, direction, and other factors.
- Virtual Personal Assistants (VPA) like Siri or Alexa or Google:– these use natural language processing to understand and respond to user requests, such as playing music, setting reminders, and answering questions.
- Autonomous vehicles – self-driving cars use AI to analyze sensor data, such as cameras and lidar, to make decisions about navigation, obstacle avoidance, and route planning.
- Fraud detection – financial institutions use AI to analyze transactions and detect patterns that are indicative of fraud, such as unusual spending patterns or transactions from unfamiliar locations.
- Image recognition – AI is used in applications such as photo organization, security systems, and autonomous robots to identify objects, people, and scenes in images.
- Natural language processing – AI is used in chatbots and language translation systems to understand and generate human-like text.
- Predictive analytics – AI is used in industries such as healthcare and marketing to analyze large amounts of data and make predictions about future events, such as disease outbreaks or consumer behavior.
- Game-playing AI – AI algorithms have been developed to play games such as chess, Go, and poker at a superhuman level, by analyzing game data and making predictions about the outcomes of moves.
Examples of Machine Learning:
Machine Learning (ML) is a subset of Artificial Intelligence (AI) that involves the use of algorithms and statistical models to allow a computer system to “learn” from data and improve its performance over time, without being explicitly programmed to do so.
Here are some examples of Machine Learning:
- Image recognition: Machine learning algorithms are used in image recognition systems to classify images based on their contents. These systems are used in a variety of applications, such as self-driving cars, security systems, and medical imaging.
- Speech recognition: Machine learning algorithms are used in speech recognition systems to transcribe speech and identify the words spoken. These systems are used in virtual assistants like Siri and Alexa, as well as in call centers and other applications.
- Natural language processing (NLP): Machine learning algorithms are used in NLP systems to understand and generate human language. These systems are used in chatbots, virtual assistants, and other applications that involve natural language interactions.
- Recommendation systems: Machine learning algorithms are used in recommendation systems to analyze user data and recommend products or services that are likely to be of interest. These systems are used in e-commerce sites, streaming services, and other applications.
- Sentiment analysis: Machine learning algorithms are used in sentiment analysis systems to classify the sentiment of text or speech as positive, negative, or neutral. These systems are used in social media monitoring and other applications.
- Predictive maintenance: Machine learning algorithms are used in predictive maintenance systems to analyze data from sensors and other sources to predict when equipment is likely to fail, helping to reduce downtime and maintenance costs.
- Spam filters in email – ML algorithms analyze email content and metadata to identify and flag messages that are likely to be spam.
- Recommendation systems – ML algorithms are used in e-commerce websites and streaming services to make personalized recommendations to users based on their browsing and purchase history.
- Predictive maintenance – ML algorithms are used in manufacturing to predict when machinery is likely to fail, allowing for proactive maintenance and reducing downtime.
- Credit risk assessment – ML algorithms are used by financial institutions to assess the credit risk of loan applicants, by analyzing data such as their income, employment history, and credit score.
- Customer segmentation – ML algorithms are used in marketing to segment customers into different groups based on their characteristics and behavior, allowing for targeted advertising and promotions.
- Fraud detection – ML algorithms are used in financial transactions to detect patterns of behavior that are indicative of fraud, such as unusual spending patterns or transactions from unfamiliar locations.
- Speech recognition – ML algorithms are used to transcribe spoken words into text, allowing for voice-controlled interfaces and dictation software.
Examples of Deep Learning:
Deep Learning is a type of Machine Learning that uses artificial neural networks with multiple layers to learn and make decisions.
Here are some examples of Deep Learning:
- Image and video recognition: Deep learning algorithms are used in image and video recognition systems to classify and analyze visual data. These systems are used in self-driving cars, security systems, and medical imaging.
- Generative models: Deep learning algorithms are used in generative models to create new content based on existing data. These systems are used in image and video generation, text generation, and other applications.
- Autonomous vehicles: Deep learning algorithms are used in self-driving cars and other autonomous vehicles to analyze sensor data and make decisions about speed, direction, and other factors.
- Image classification – Deep Learning algorithms are used to recognize objects and scenes in images, such as recognizing faces in photos or identifying items in an image for an e-commerce website.
- Time series forecasting – Deep Learning algorithms are used to forecast future values in time series data, such as stock prices, energy consumption, and weather patterns.
Work in AI Engineer vs ML Engineer vs DL Engineer
Working in AI is not the same as being an ML or DL engineer. Here’s how you can tell those careers apart and decide which one is the right call for you.
What Does an Artificial Intelligence Engineer Do?
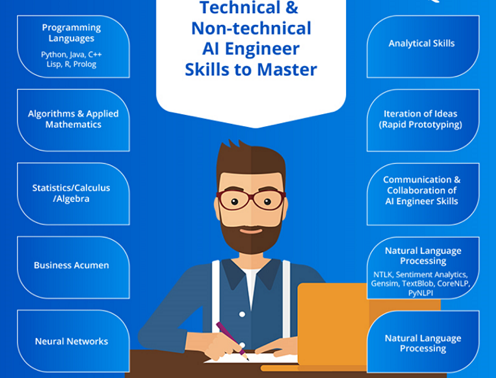
An AI Engineer is a professional who designs, develops, and implements artificial intelligence (AI) systems and solutions. Here are some of the key responsibilities and tasks of an AI Engineer:
- Design and development of AI algorithms: AI Engineers design, develop, and implement AI algorithms, such as decision trees, random forests, and neural networks, to solve specific problems.
- Data analysis: AI Engineers analyze and interpret data, using statistical and mathematical techniques, to identify patterns and relationships that can be used to train AI models.
- Model training and evaluation: AI Engineers train AI models on large datasets, evaluate their performance, and adjust the parameters of the algorithms to improve accuracy.
- Deployment and maintenance: AI Engineers deploy AI models into production environments and maintain and update them over time.
- Collaboration with stakeholders: AI Engineers work closely with stakeholders, including data scientists, software engineers, and business leaders, to understand their requirements and ensure that the AI solutions meet their needs.
- Research and innovation: AI Engineers stay current with the latest advancements in AI and contribute to the research and development of new AI techniques and algorithms.
- Communication: AI Engineers communicate the results of their work, including the performance of AI models and their impact on business outcomes, to stakeholders.
An AI Engineer must have a strong background in computer science, mathematics, and statistics, as well as experience in developing AI algorithms and solutions. They should also be familiar with programming languages, such as Python and R.
What Does a Machine Learning Engineer Do?
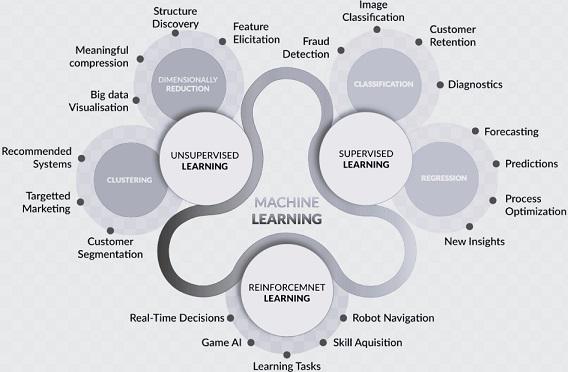
A Machine Learning Engineer is a professional who designs, develops, and implements machine learning (ML) systems and solutions. Here are some of the key responsibilities and tasks of a Machine Learning Engineer:
- Design and development of ML algorithms: Machine Learning Engineers design, develop, and implement ML algorithms, such as decision trees, random forests, and neural networks, to solve specific problems.
- Data analysis: Machine Learning Engineers analyze and interpret data, using statistical and mathematical techniques, to identify patterns and relationships that can be used to train ML models.
- Model training and evaluation: Machine Learning Engineers train ML models on large datasets, evaluate their performance, and adjust the parameters of the algorithms to improve accuracy.
- Deployment and maintenance: Machine Learning Engineers deploy ML models into production environments and maintain and update them over time.
- Collaboration with stakeholders: Machine Learning Engineers work closely with stakeholders, including data scientists, software engineers, and business leaders, to understand their requirements and ensure that the ML solutions meet their needs.
- Research and innovation: Machine Learning Engineers stay current with the latest advancements in ML and contribute to the research and development of new ML techniques and algorithms.
- Communication: Machine Learning Engineers communicate the results of their work, including the performance of ML models and their impact on business outcomes, to stakeholders.
A Machine Learning Engineer must have a strong background in computer science, mathematics, and statistics, as well as experience in developing ML algorithms and solutions. They should also be familiar with programming languages, such as Python and R, and have experience working with ML frameworks and tools.
What Does a Deep Learning Engineer Do?
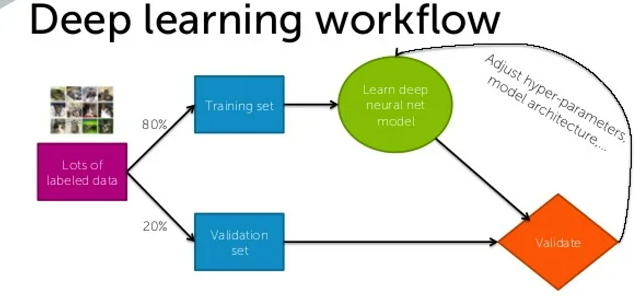
A Deep Learning Engineer is a professional who designs, develops, and implements deep learning (DL) systems and solutions. Here are some of the key responsibilities and tasks of a Deep Learning Engineer:
- Design and development of DL algorithms: Deep Learning Engineers design, develop, and implement deep neural networks and other DL algorithms to solve specific problems.
- Data analysis: Deep Learning Engineers analyze and interpret large datasets, using statistical and mathematical techniques, to identify patterns and relationships that can be used to train DL models.
- Model training and evaluation: Deep Learning Engineers train DL models on massive datasets, evaluate their performance, and adjust the parameters of the algorithms to improve accuracy.
- Deployment and maintenance: Deep Learning Engineers deploy DL models into production environments and maintain and update them over time.
- Collaboration with stakeholders: Deep Learning Engineers work closely with stakeholders, including data scientists, software engineers, and business leaders, to understand their requirements and ensure that the DL solutions meet their needs.
- Research and innovation: Deep Learning Engineers stay current with the latest advancements in DL and contribute to the research and development of new DL techniques and algorithms.
- Communication: Deep Learning Engineers communicate the results of their work, including the performance of DL models and their impact on business outcomes, to stakeholders.
Working Engineer in AI vs ML vs DL: Is There a Difference?
Yes, there are differences between working as an engineer in AI (Artificial Intelligence), ML (Machine Learning), and DL (Deep Learning), although they are closely related fields. Here's an overview of the distinctions:
AI Engineer:
- Role and Focus: AI engineers work on developing systems that can perform tasks that typically require human intelligence. This can include a wide range of technologies, not limited to machine learning and deep learning. AI engineers work on creating intelligent agents that can reason, plan, learn, and perceive their environment.
- Tasks: They design algorithms and systems for natural language processing, robotics, computer vision, knowledge representation, and more.
- Skills: Strong problem-solving skills, expertise in various AI techniques, familiarity with knowledge representation, and a broad understanding of AI concepts.
ML Engineer:
- Role and Focus: Machine learning engineers concentrate on building systems that can learn from data without being explicitly programmed. They focus on creating models that improve their performance over time as they encounter more data.
- Tasks: Data preprocessing, feature engineering, model selection, training, and deployment. ML engineers work on various types of machine learning algorithms, including traditional techniques and deep learning.
- Skills: Proficiency in statistics, data analysis, programming languages like Python, and knowledge of machine learning algorithms and frameworks.
DL Engineer:
- Role and Focus: Deep learning engineers specialize in creating and implementing deep neural networks, a subset of machine learning algorithms inspired by the structure of the human brain. Their primary focus is on complex architectures and large datasets.
- Tasks: Designing and training deep neural networks, often using frameworks like TensorFlow and PyTorch. They may work on tasks such as image recognition, natural language processing, and speech synthesis.
- Skills: In-depth knowledge of neural network architectures, understanding of backpropagation, experience with deep learning frameworks, and expertise in working with large-scale datasets.
- Strong problem-solving and analytical skills.
- Understanding of various AI techniques and algorithms.
- Familiarity with knowledge representation and reasoning.
- Ability to work on complex and interdisciplinary projects.
- Proficiency in programming languages like Python, Java, or C++.
- Creative thinking to design innovative AI solutions.
- Opportunity to work on diverse AI applications.
- Involvement in cutting-edge research and development.
- Versatility in tackling various AI challenges.
- Need to keep up with a broad range of AI techniques and advancements.
- Strong background in mathematics, statistics, and data analysis.
- Proficiency in programming languages like Python and libraries like scikit-learn, TensorFlow, and PyTorch.
- Data preprocessing and feature engineering expertise.
- Knowledge of various machine learning algorithms and their applications.
- Ability to evaluate model performance and fine-tune hyperparameters.
- High demand in industries for implementing machine learning solutions.
- Opportunity to work with diverse datasets and domains.
- Potential to solve real-world problems through predictive modeling.
- Need to stay updated with rapidly evolving machine learning techniques and tools.
- In-depth understanding of neural network architectures and backpropagation.
- Proficiency in deep learning frameworks like TensorFlow, Keras, and PyTorch.
- Expertise in working with GPUs for efficient model training.
- Ability to design and fine-tune deep neural network architectures.
- Knowledge of data augmentation techniques for improving model performance.
- Involved in cutting-edge advancements in deep learning.
- High demand for expertise in complex neural network models.
- Opportunities in fields like computer vision, NLP, and autonomous systems.
- Requires a deep understanding of complex neural network architectures.
- Demand for skilled DL engineers might be higher in certain industries compared to others.
Comments
Post a Comment